Current Developments in Interpretable Machine Learning
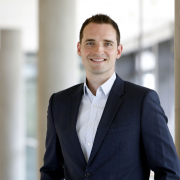
Speaker: Prof. Dr. Patrick Zschech
Abstract: Most machine learning (ML) models are based on complex mathematical operations and therefore do not offer the possibility of fully understanding how the models work. Due to such black-box properties, these models are difficult to verify and are therefore unsuitable for many areas of application, such as finance, healthcare, and law. To this end, Patrick Zschech will provide an overview of current developments in the field of interpretable machine learning. Among other things, he shows that (i) modern ML models do not necessarily have to be opaque models in order to achieve high predictive performance, that (ii) in addition to algorithmic developments, it is important to focus on user-centered needs, and that (iii) linking interpretable ML models with generative technologies is a promising direction, but also involves unexpected risks.
Short Bio: Patrick Zschech is Professor for Intelligent Information Systems & Processes at Leipzig University, Germany. Previously, he was Assistant Professor at FAU Erlangen-Nürnberg, where he headed a BMBF junior research group on white-box AI together with Prof. Dr. Mathias Kraus. Patrick received his Ph.D. in Business Information Systems from Technische Universität Dresden. His research focuses on business analytics, machine learning, and artificial intelligence, with particular emphasis on the design, analysis, and deployment of intelligent information systems. His results have been published in various IS and OR journals such as European Journal of Operational Research, Health Care Management Science, Decision Support Systems, Business & Information Systems Engineering, and Electronic Markets, and presented at international conferences such as ICIS, ECIS, and WI.