Chair of Data Science: Machine Learning and Data Engineering (Prof. Gieseke)
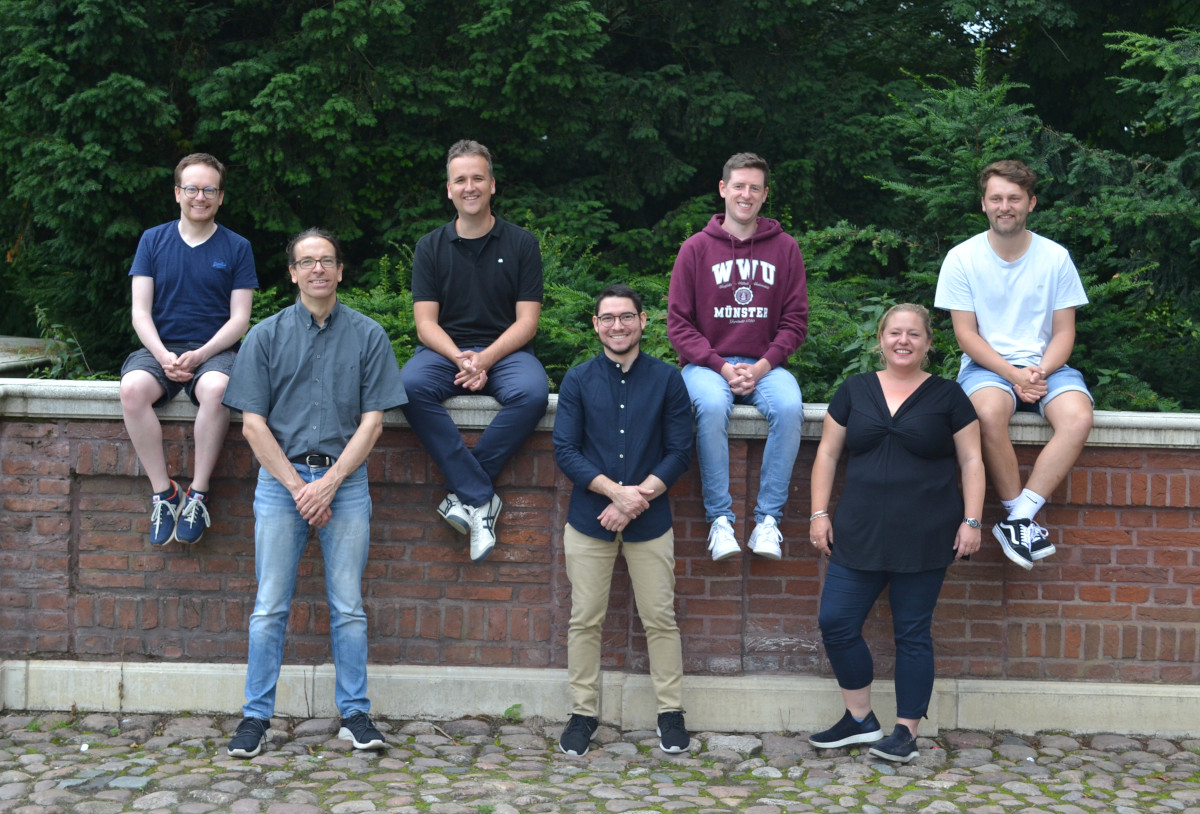
The data volumes have increased dramatically in various domains over recent years. This is the case, for instance, in remote sensing, where satellites produce data volumes in the petabyte range per year. A similar "data flood" can be observed in many other disciplines as well including medicine, social media, finance, or in the context of modern energy systems. The research focus of the Machine Learning and Data Engineering group is on the development of efficient and scalable implementations for modern machine learning models. In particular, we make use of high-performance computing (e.g. GPGPU programming) and distributed computing to reduce the practical runtime needed for the overall data analysis. In many cases, the original algorithmic building blocks are not suited for modern hardware architectures. The adaptation of known and the development of new methods that can efficiently deal with huge amounts depicts one of the main activities of our group.